About the project
Our vision of the future
Predictive personalized Modeling
Post-myocardial infarct patients have an increased risk for scar-based ventricular tachycardia (VT), an abnormal heart rhythm, which may lead to sudden cardiac death (SCD). Ablation therapy is used to treat VTs, but has moderate success rates of 50- 80%. Secondly, an implanted cardioverter defibrillator (ICD) can help prevent SCD if ablation is inexecutable. Current guidelines for ICD treatment are based on left ventricular ejection fraction but prove to be insufficient in identifying patients with a high risk of VT. The electro-mechanical interaction of the heart is key to understand VT development. Here, patient-specific computational models can be used to create a cardiac Digital Twin which can serve as a decision support tool in the clinic.
Our project uses Data Integration on patient data & history, for Patient-specific IGA modeling, Electro-mechanically coupled modeling and Artificial Intelligence modeling. The resulting decision model can be used to improve the understanding and prediction of the occurrence of Ventricular Tachycardias and provide optimized patient-specific treatment using computationally efficient models.
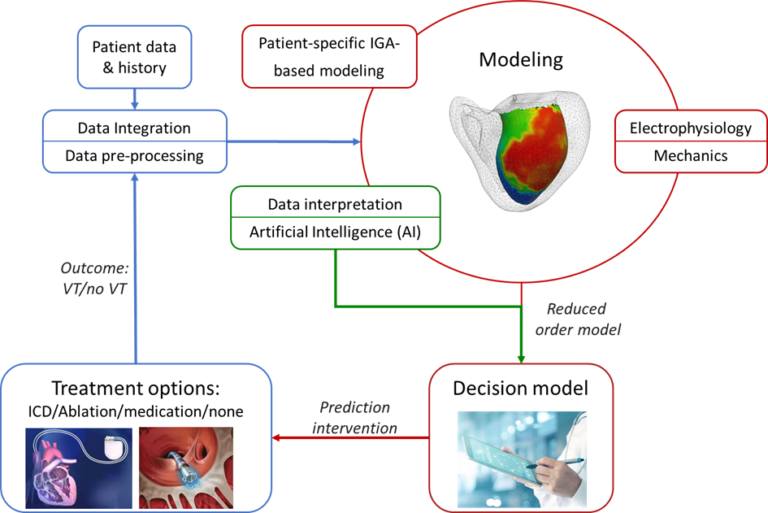
Data integration & Pre-processing
Melissa Niemantsverdriet
Marloes de Winter
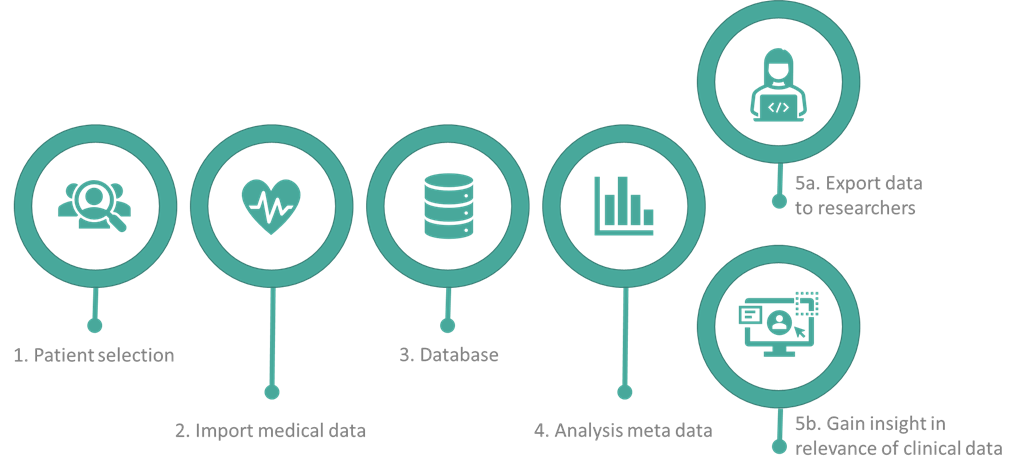
The linking pin between the technological research performed by the PhD students and the clinical application: A clinical workflow is developed in order to acquire patient-specific data. In this workflow, the data is semi-automatically obtained, analyzed and processed to be used for the physiological and data models of the COMBAT-VT project. This workflow has five essential components:
- Selection of patients;
- Obtaining the data from the different information systems at the hospital;
- Saving data according to the FAIR principles;
- Analyzing the data to explore what is exactly saved from the patients and what is useful for the models;
- Preprocessing the data and sharing the data with the PhD researchers working at the TU/e and providing insight with a clinical data interface.
Patient-specific IGA-based modeling
To study potential mechanisms involved in VTs, new methods are required that enable high-fidelity analyses in a computationally
efficient and accurate manner. Additionally, incorporating these methods into the clinical workflow should allow for the rapid output of indicators that support clinical decision-making. We aim to achieve the goals by: (i) developing a computationally robust, efficient, and accurate framework that provides a basis to investigate potential mechanisms of VTs, subject to varying input, particularly regarding geometric data. Use is made of the Isogeometric Analysis (IGA) paradigm, to assess whether it offers significant benefits compared to the traditional finite element (FE) approach. (ii) Developing a reduced-order model that limits computation costs, while providing quantitative indicators of the cardiac behavior accompanied by a measure of uncertainty. In doing so, we leverage the IGA-based full-order model results to train and enrich the parameters of an established semi-analytical model using Bayesian inference. It interpolates new parameter values using Gaussian Processes. Once properly trained, the reduced-order model provides a cardiac response within specified uncertainty
bounds in a fraction of the time required for the full-order simulations.
The developed methods enhance the ability to test new hypotheses in cardiac mechanics, particularly those that require multiple computations or the incorporation of additional physics. This accelerates computational research of various disease treatments, ultimately benefiting clinical research. Furthermore, the developed framework can be extended to more advanced cardiac models and, in principle, is also applicable to other engineering fields.
Robin Willems
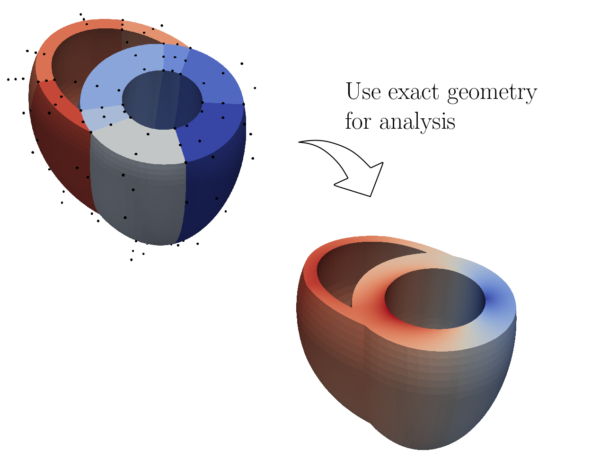
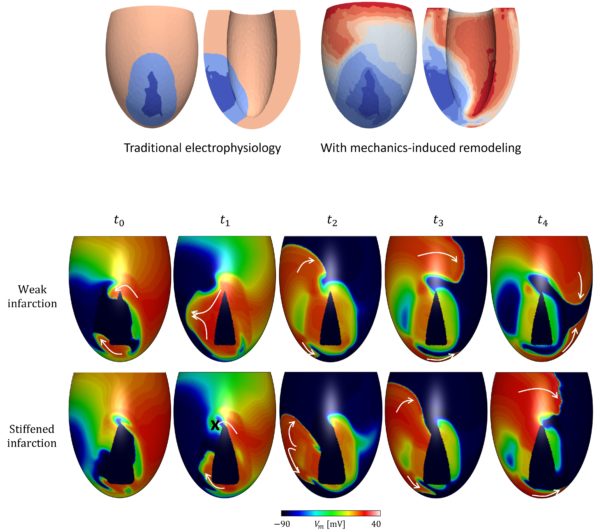
Electro-mechanical modeling
In traditional electrophysiology models, remodeling of electrical properties is taken into account at the infarct area only. In our project, information from mechanical simulations is considered too by implementing conduction slowing in areas with abnormal mechanics, which can extend beyond the structural infarct area. The hypothesis that strain affects connexin density, and therewith conductivity, is central to this project. It results in a more heterogeneous substrate for ventricular tachycardia. We use this hypothesis to test whether in can explain the long time frame between infarction and the onset of VT (sometimes up to several years), by simulating infarct stiffening to obtain strains corresponding to the chronic infarct state. Furthermore, the effects of the hypothesis on ablation therapy success is explored.
Evianne Willems
Artificial intelligence modeling
Currently, physicians mainly focus on the ejection fraction to determine whether a patient has a high risk of developing VTs. However, this measurement has shown to be suboptimal in identifying these patients. A lot of data (among others, echo, MRI, ECG and lab values) of these patients is available in the hospital. We hypothesize that there are subtle changes in these measurements over time which the physicians are unaware of that might be better at predicting VTs. To unravel these possible predictors, data-driven models like Artificial Intelligence (AI) can play a major role. AI is known for its ability to find hidden structures in data without predefined knowledge, which is exactly what is needed here. However, they are hampered by noisy and missing data and fall short in their inter- and extrapolating capabilities.
Besides patient data there is also physiological and physical knowledge available in the form of computer models (projects of Evianne and Robin). These physics-based models only use a small amount of the available data, cannot directly include risk factors, need patient-specific parameter tuning and can only be used if the physics are known. Nevertheless, they are vital to gain insight in disease development, treatment planning, and to calculate parameters which cannot be excessed clinically.
This subproject focusses on developing a VT Digital Twin using a hybrid modelling approach. A Digital twin is a predictive computer model which dynamically pairs the physical and digital worlds to represent (part of) a patient and is continuously updated with new data. Importantly, this digital twin should be able to evolve over time.
In this project, we develop an unique retrospective dataset, collecting multimodal data from post-infarction patients over time. Some patients developed VT, while others served as a control group. Next, we investigate if temporal changes could be predictive of VT with both statistical models and AI models. This information can help us determine which data could be of use for our Digital Twin. In the final stage we develop a proof of concept of how a possible Digital Twin which evolve over time could look like.
Carlijn Buck